Long essay today; let’s get straight to it. (Note — you might have a better time with footnotes on web/app than email, if that matters to you.)
Nature by Alan Warburton, CC 4.0By Jessica Dai
Credulous, breathless coverage of “AI existential risk” (abbreviated “x-risk”) has reached the mainstream. Who could have foreseen that the smallcaps onomatopoeia “ꜰᴏᴏᴍ” — both evocative of and directly derived from children’s cartoons — might show up uncritically in the New Yorker? More than ever, the public discourse about AI and its risks, and about what can or should be done about those risks, is horrendously muddled, conflating speculative future danger with real present-day harms, and, on the technical front, confusing large, “intelligence-approximating” models with algorithmic and statistical decision-making systems.
What, then, are the stakes of progress in AI? For all the pontification about cataclysmic harm and extinction-level events, the current trajectory of so-called “alignment” research seems under-equipped — one might even say misaligned — for the reality that AI might cause suffering that is widespread, concrete, and acute. Rather than solving the grand challenge of human extinction, it seems to me that we’re solving the age-old (and notoriously important) problem of building a product that people will pay for. Ironically, it’s precisely this valorization that creates the conditions for doomsday scenarios, both real and imagined.
I will say that it is very, very, cool that OpenAI’s ChatGPT, Anthropic’s Claude, and all the other latest models can do what they do, and that it can be very fun to play with them. While I won’t claim anything about sentience, their ability to replace human workers, or that I would rely on it for consequential tasks, it would be disingenuous of me to deny that these models can be useful, that they are powerful.
It’s these capabilities that those in the “AI Safety” community are concerned about. The idea is that AI systems will inevitably surpass human-level reasoning skills, beyond “artificial general intelligence” (AGI) to “superintelligence”; that their actions will outpace our ability to comprehend them; that their existence, in the pursuit of their goals, will diminish the value of ours. This transition, the safety community claims, may be rapid and sudden (“ꜰᴏᴏᴍ”). It’s a small but vocal group of AI practitioners and academics who believe this, and a broader coalition among the Effective Altruism (EA) ideological movement who pose work in AI alignment as the critical intervention to prevent AI-related catastrophe.
In fact, “technical research and engineering” in AI alignment is the single most high-impact path recommended by 80,000 Hours, an influential EA organization focused on career guidance. In a recent NYT interview, Nick Bostrom — author of Superintelligence and core intellectual architect of effective altruism — defines “alignment” as “ensur[ing] that these increasingly capable A.I. systems we build are aligned with what the people building them are seeking to achieve.”
Who is “we”, and what are “we” seeking to achieve? As of now, “we” is private companies, most notably OpenAI, the one of the first-movers in the AGI space, and Anthropic, which was founded by a cluster of OpenAI alumni. OpenAI names building superintelligence as one of its primary goals. But why, if the risks are so great? In their own words:
First, we believe it’s going to lead to a much better world than what we can imagine today (we are already seeing early examples of this in areas like education, creative work, and personal productivity)… economic growth and increase in quality of life will be astonishing.
Second, we believe it would be unintuitively risky and difficult to stop the creation of superintelligence. Because the upsides are so tremendous, the cost to build it decreases each year, the number of actors building it is rapidly increasing, and it’s inherently part of the technological path we are on… we have to get it right.
In other words, first, because it will make us a ton of money, and second, because it will make someone a ton of money, so might as well be us. (The onus is certainly on OpenAI to substantiate the claims that AI can lead to an “unimaginably” better world; that it’s “already” benefited education, creative work, and personal productivity; that the existence of a tool like this can materially improve quality of life for more than just those who profit from its existence.)
Of course, that’s the cynical view, and I don’t believe most people at OpenAI are there for the sole purpose of personal financial enrichment. To the contrary, I think the interest — in the technical work of bringing large models into existence, the interdisciplinary conversations of analyzing their societal impacts, and the hope of being a part of building the future — is genuine. But an organization’s objectives are ultimately distinct from the goals of the individuals that comprise it. No matter what may be publicly stated, revenue generation will always be at least a complementary objective by which OpenAI’s governance, product, and technical decisions are structured, even if not fully determined. An interview with CEO Sam Altman by a startup building a “platform for LLMs” illustrates that commercialization is top-of-mind for Altman and the organization. OpenAI’s “Customer Stories” page is really no different from any other startup’s: slick screencaps and pull quotes, name-drops of well-regarded companies, the requisite “tech for good” highlight.
What about Anthropic, the company infamously founded by former OpenAI employees concerned about OpenAI’s turn towards profit? Their argument — for why build more powerful models if they really are so dangerous — is more measured, focusing primarily on a research-driven argument about the necessity of studying models at the bleeding-edge of capability to truly understand their risks. Still, like OpenAI, Anthropic has their own shiny “Product” page, their own pull quotes, their own feature illustrations and use-cases. Anthropic continues to raise hundreds of millions at a time.
So OpenAI and Anthropic might be trying to conduct research, push the technical envelope, and possibly even build superintelligence, but they’re undeniably also building products — products that carry liability, products that need to sell, products that need to be designed such that they claim and maintain market share. Regardless of how technically impressive, useful, or fun Claude and GPT-x are, they’re ultimately tools (products) with users (customers) who hope to use the tool to accomplish specific, likely-mundane tasks.
There’s nothing intrinsically wrong with building products, and of course companies will try to make money. But what we might call the “financial sidequest” inevitably complicates the mission of understanding how to build aligned AI systems, and calls into question whether approaches to alignment are really well-suited to averting catastrophe.
In the same NYT interview about the possibility of superintelligence, Bostrom — a philosopher by training, who, as far as anyone can tell, actually has approximately zero background in machine learning research — says of alignment: “that’s a technical problem.”
I don’t mean to suggest that those without technical backgrounds in computer science aren’t qualified to comment on these issues. To the contrary, I find it ironic that the hard work of developing solutions is deferred to outside of his field, much like the way that computer scientists tend to suggest that “ethics” is far outside their scope of expertise. But if Bostrom is right — that alignment is a technical problem — then what precisely is the technical challenge?
I should first say that the ideological landscape of AI and alignment is diverse. Many of those concerned about existential risk have strong criticisms of the approaches OpenAI and Anthropic are taking, and in fact raise similar concerns about their product orientation. Still, it’s both necessary and sufficient to focus on what these companies are doing: they currently own the most powerful models, and unlike, say, Mosaic or Hugging Face, two other vendors of large models, take alignment and “superintellig
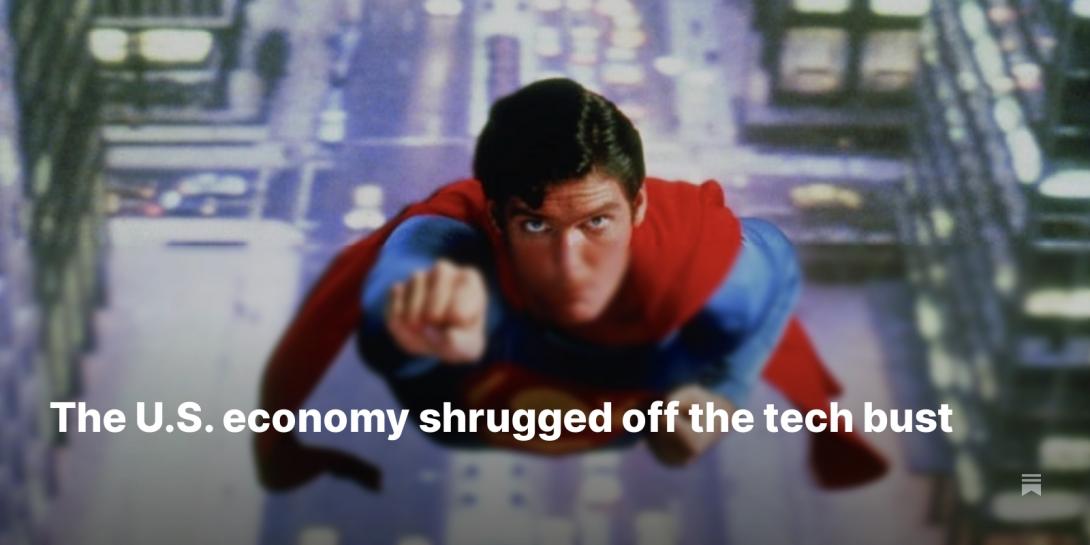
Feeds item